M^3Check: A Demonstration System for Multilingual Multi-clue Multi-Hop Fact-Checking
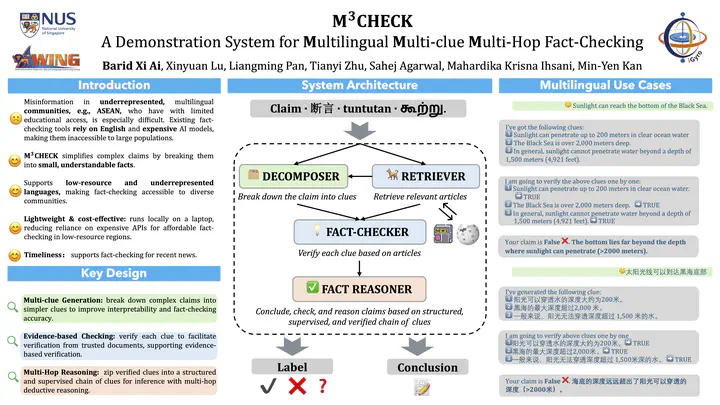
Imagine that, while we cannot simply Google a complex claim, we can still find some relevant clues to help us. The question is, how can we use these clues to verify the input claim? M^3Check is a RAG model that follows three major steps: 1) break down the claim into clues, 2) verify each clue based on articles, and 3) conclude, check, and reason the claim based on a structured, supervised, and verified chain of clues. This project leverages findings from multiple projects, offering a good example of how research outcomes can be applied to address a real-world problem.